
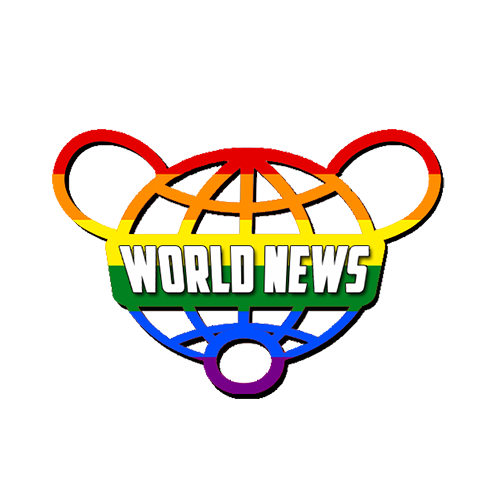
This is a problem that’s becoming outdated, thanks to NIH now requiring females to be included in studies in order to receive grant funding–barring an exceptional reason for studying males alone (e.g., male-specific problems). They are even requiring cell lines for in vitro studies to be derived, at least in part, from females, rather than from males alone.
The best time to start was decades ago, but at least they’ve started.